Katholieke Universiteit Leuven
Architectural Engineering Division
Prof. Dr.-Ing. Philipp Geyer
For reducing energy consumption and emissions of the built environment, it is vital to manage building stocks and develop strategies for energy efficiency and building-integrated renewable energy supply. However, the development of such strategies for hundred to 10,000 buildings is a major challenge. Therefore, the research project develops methods to cluster buildings based on their sensitivity to energy efficiency measures and to identify their intelligent interconnection by thermal microgrids and other smart local energy systems.
First, the groups derived by algorithmic clustering allow an easy development of tailored retrofit strategies. The clustering is based on the reduction of CO2 emission per investment cost unit for each retrofitting measure. The better fitting of retrofit measures and strategies to clusters in contrast to the conventional classification based on building type and age is demonstrated. For these groups, an easy development of retrofit strategies is possible.
Furthermore, district heating systems have proven to supply heat for buildings both energy and cost efficient. Thermal microgrids can be understood as a special form of district heating: local, small scale, bi-directional and supplied by different thermal sources that are connected in a network. Given a suitable combination of loads, number and distance of buildings they offer ecologic and economic advantages compared to individual heating systems. A novel method using fuzzy logic as data analysis techniques on geo-referenced building data to identify suitable configurations of buildings yields efficient and effective thermal microgrids. For the identification both non-spatial and spatial data from a database are combined to form a set of selection criteria. The method is also applicable for the identification of electric smart grids or other similar network types.
Both methods are applied to the data of the research project Zernez Energia 2020, which deals with a Swiss village to become carbon neural. The methods served in Zernez to develop a cost-effective retrofit strategy saving 80% of CO2 emissions.
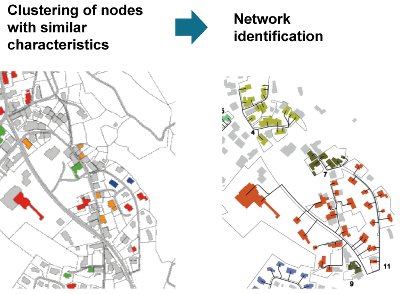